Businesses should focus on building strong customer relationships through innovative marketing channels. Rajkumar Venkatesan discusses how entrepreneurs can incorporate AI into their marketing strategies. In this episode, he joins Dr. Diane Hamilton as they dive deep into understanding our customers through marketing communication. Raj has written about and taught quantitative marketing to MBA and executive education students worldwide. He has taught courses on marketing analytics and marketing technology products for five years. His evident fascination with marketing and business is the best way to help us stay motivated in our paths and deliver superior customer experiences. This episode is for all who want to advance in their careers and take the lead in their professional journeys.
I’m glad you joined us because we have Rajkumar Venkatesan. Raj is a Professor of Business Administration at Darden. He’s also the author of a new book, The AI Marketing Canvas. He has been published extensively in The Journal of Marketing, HBR, International Journal of Marketing Research, you name it. His book is helpful for explaining the value of AI and how marketing has evolved. It’s going to be a great discussion.
—
Watch the episode here
Listen to the podcast here
The AI Marketing Canvas: A Five-Stage Road Map To Implementing Artificial Intelligence In Marketing With Rajkumar Venkatesan
I am here with Rajkumar Venkatesan who is a Professor of Business Administration at Darden Business School at the University of Virginia. He’s written so much. I’m excited about his book, The AI Marketing Canvas. It’s nice to have you here, Raj.
It’s a pleasure to be here, Diane. Thanks for having me.
You’re welcome. I was looking forward to this. I have a lot of marketing courses I teach. We’re going to have plenty to chat about. I want to get a backstory on you. You’ve been published extensively. You named the prestigious journal and you’re in it, from HBR and anything else that you could think of that would be considered as well. How did you reach this level? Did you always have a fascination with marketing and business? Give me your backstory.
Thank you for the kind introduction, Diane. I started with my undergrad degree in Computer Engineering. I completed it in ‘97 in India. I talked about Computer Engineering because that has influenced what I did in marketing ever since. In ‘97, I distinctly remember in my final year of Engineering, I took classes in neural networks and did my final year project. We did this semester-long project in genetic algorithms. Those two things are part of the AI toolkit that we all talked about now.
After my Engineering, I came to the United States to do my PhD in Marketing. My PhD advisor told me at the time that technology and computers are going to play a big role in marketing, and asked if I would be interested in specializing in that for my PhD. At that time, I didn’t think much of it. I said, “Yes. I’ll do it.” I took classes in economics, marketing, consumer behavior, and all of those were fascinating for me. I had this technology background and these classes told me where they can be applied. It was fascinating to see the combination of those.
I stuck around and worked on this in my PhD. My first job was at the University of Connecticut. I taught marketing research there. I started at UVA at Darden in 2006 teaching marketing analytics there. At that time, it was still new. Each year, I saw the students increase in the elective and the interest kept increasing. In 2015, I started teaching digital marketing also. Darden was life-changing. Around that time is when I started to understand marketing and learn about the practice of marketing. Our focus on case studies helped me work with companies and exec education. All that gave me a perspective on how the world of marketing is changing.
My first book was Marketing Analytics. That was based on my course at that time. It was a tools book. It was a book on how to use analytics. As I was working on this book, I realized that’s great but where there really is a need is for rethinking the marketing process and the way technology, data and algorithms are changing marketing at the CMO and marketing leader level. Jim, my co-author, used to do some guest lectures at Darden at the time. He was thinking about the same things but from a different vantage point with his practical experience, leading the sales division at Google.
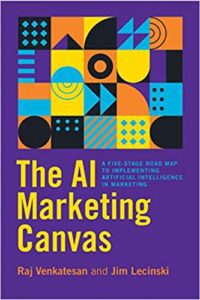
Over many conversations, it evolved into us deciding to write this book on AI and marketing to put together the perspective on how leaders like marketing leaders can use AI in their marketing strategy. It’s been a great ride. In ‘97, I didn’t know where it’ll all end up. I’ve seen the engineering and marketing worlds collide. It’s been great to be a part of it. Also, I’ve been fortunate to be able to do my part in making this happen.
There’s a lot that you said there that is interesting. When I was the MBA program chair at the Forbes School of Business, which is part of the University of Arizona’s Global Campus now, I did some research and work with Bruce Rogers. He had created this Publish Or Perish report for Forbes at the time. We created a lot of different content. They had me write a couple of different brand publishing courses that I was to present at the CMO Forbes Summits and things like that. It was such a challenge in many ways.
I’ve taught a lot of marketing classes. What I found interesting about that Publish Or Perish report that Bruce had worked on at the time was he went to all the CMOs and asked them, “What software and different things are you using? What did you like?” Bruce Rogers has been on the show and he’s a great guest. It’s a report that gives you an idea of what works for everybody and how they communicate in all these different software systems.
It showed me how complicated it is out there to be in marketing, and that they don’t all connect and they don’t all tell the other systems how they are working. You need to have this analytics aspect of everything. There was a lot to be still created at that point to connect everything. The biggest challenge I kept hearing was they were trying to come up with messages to reach people that felt personalized, but they were trying to do it at scale. Is that still a real challenge for people? How is AI impacting that?
It is still a challenge. AI is certainly bringing us closer to that goal. I remember around the same time as this report, there was the whole idea of one-on-one marketing. I remember Peppers & Rogers had this book on database marketing as it was called at that time. This was an evolution from TV to cables to segmented marketing to database marketing. It was the mid-‘90s when CRM databases and Siebel Systems were all coming up. We were looking at direct mail and emails at that time and we’re thinking about personalization.
You’re right that the challenge, and we talked about this in the book, is about how it’s the foundation of data that is going to enable all of this to happen. As we have gotten richer information on consumers, we’ve been able to do more and more in marketing. The raw material is the data on how well we understand consumers. With direct mail, we started learning about individual consumers and identifying them, and being able to target them better. The digital, the email, the display, the Web 2.0 and social media have gotten a little better.
What happened with AI is that we reached a level in both data and algorithms that are helping us personalized even more. I’ll go back to the purchase funnel. We have AIDA, Awareness, Interest, Desire, and Action. Until Web 2.0, we only knew about customers until they bought. That’s why the purchase funnel stops at the action when they buy something. We don’t know about the customers until they come back to buy. We don’t know anything about how they’re using the product. We don’t know anything about how they’re talking about their product with their friends. All of that started to change. We got an insight into that.
With social media, we got an insight into how they’re talking about it with their friends. With AI and with the new smart systems, we are able to see how people are using the product. That changes the relationship between marketers and consumers. We have a much better understanding of how people use things. That allows us to make some customizations and also allows us to provide more personalized recommendations and marketing communications. We’ve had better algorithms and better data, but from a marketer’s perspective, it’s this whole new world of consumption that you are able to look into that is helping and changing your marketing.
In your book, you start by explaining what AI is and how it’s different from traditional computer science approaches. Can you give a little background on that for people who are not exactly sure what you mean by AI in this context?
[bctt tweet=”If you’re starting fresh in a business, don’t give up. Every successful company right now has started somewhere. ” via=”no”]Whenever I talk about this, I first ask the people when I do webinars, “What is AI?” If you have 100 people, you get 100 definitions.
It’s like, “What’s technology?” It’s hard to explain what it is.
We struggled with this a lot in terms of, how do we define this? One of the things that we went back to was there was this definition of things that a machine does. If a human does it, it would be considered intelligent. It’s a little broad and abstract but it is saying that if my friend tells me, “You should watch this new movie.” That means my friend knows me and my friend is doing something intelligent about his knowledge about me. If a machine does it, then that’s AI.
I’m seeing the if-then thing in the code in my mind.
What the algorithm changed dramatically for marketers at least was when you have these if-then-else, it’s a difficult thing. To our mind, Google Images was the biggest scalable AI implementation that is relevant for marketing. In Google Images, the main challenge ends up being a silly thing. How do you differentiate between a muffin and a cat? If you go with if-then-else statements for a muffin, you can say, “It’s a brown circle with three red black dots in it.” That’s the same thing that describes a cat. You can say, “There are whiskers around the center dot.” You can see some lines around the muffin too and they’re around one of the dots.
These external statements start breaking down very rapidly. There are many special cases that they break down and you keep adding these. They become complex and that’s why we never use them. What changed with AI was that it’s now something that is not a buzz, it’s here to stay. We moved from external statements to starting about data. We said, “Let’s feed the machine all images and information that is first coded by humans to say, ‘This is a muffin and this is a cat.’” The machines are good with neural nets and deep learning AI algorithms. They can learn these rules themselves.
When they’re predicting, they’re not saying, “This is a muffin or a cat,” They are giving probabilities. They’re saying, “This is more like a cat,” which is how humans behave when they’re confused between two pictures. They take a guess and they have confidence in their guesses. That changed the whole dynamic about us being able to use AI for scalable operations, image recognition, video recognition, the voice of the customer, and text analysis. If-then-else statements have been long discarded and now we are more about, “Let’s feed the machine data to train it.” It became more a data science paradigm than a rules paradigm.
It’s fascinating because I haven’t done any programming since I programmed my Calculus homework in the early ‘80s. It’s been a long time, let’s put it that way. I have a good concept of what you’re talking about. The book is called The AI Marketing Canvas. You have a five-stage framework for implementing what you termed as the AI Marketing Canvas. First of all, what do you mean by AI Marketing Canvas? I want to talk about that five-stage framework.
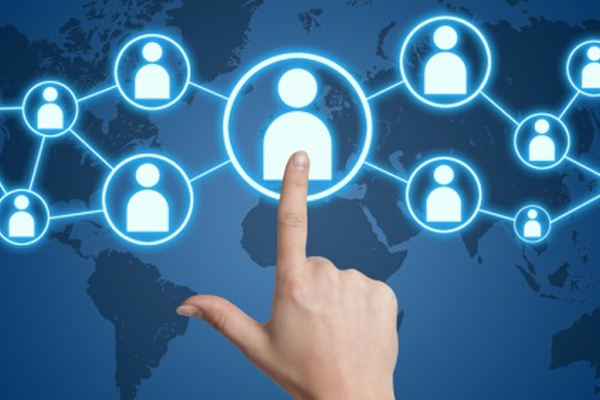
One of the things we found in writing the book is that the biggest challenge for using AI in marketing, and surveys after surveys had shown this at that time, is the senior leaders didn’t understand how to include this into a strategic vision for marketing. We have all this nice new technology. We hear about these cool things that are going on. What does it mean for us as marketers in our brands? That’s where we began defining, “As a CMO, what are you supposed to do with this?”
Our goal with AI that we propose is that marketers keep doing what they’re doing, delivering superior customer experiences but using AI data and algorithms to personalize this experience. We define customer experience and engagement consisting of four phases, acquisition, retention, growth and customer advocacy or word of mouth. Eventually, that becomes the goal of personalizing all aspects of customer engagement.
The canvas gives you a roadmap to where you begin on how you get to this goal. We like the Business Model Canvas, which is popular. We wanted to do something like that for marketing because we saw how useful it is for business models and strategy. That’s why we borrowed the term canvas and we laid it out on one page, how can you think about these five stages with the eventual goal of personalizing every aspect of the customer engagement?
You’re touching on many things that I’ve talked about on this show about the challenges in marketing. I’m teaching an entrepreneurship course where they’re creating their ideas. When you talk to them about the marketing things, they go, “I just get on social media.” They don’t go into too much depth because they don’t see how challenging it can be. Some of the big brands and the big campaigns will even put a lot of time and effort into their messages. Some of them haven’t been super successful. Some people say, “Any publicity is good publicity.” Let’s look at Gillette’s campaign about men, the “They could do better” thing. When companies have some situations like that where the message falls flat or they upset people as they did with that campaign, haven’t they gone through quite a bit of testing of this? Don’t they have that quantifiable information before they launch these campaigns or did they miss the boat on doing that?
First of all, I don’t know the internal details of how this would have happened. I can get a lot of people to experiment and I’m sure all the big brands do that. The challenge ends up being is that the data, if it is very clear, then it’s helpful. The challenge is it’s not always data plus inclusion. The data is a little uncertain. There are questions about sampling. You cannot sample everybody. There are many different things that go into deciding eventually whether to launch a campaign or not.
What you see in the outcome and the metrics you’ve looked at could also be something where you’re looking at short-term metrics mostly. Also, maybe some of these are long-term outcomes that you were not able to predict when you are doing your experimentation. That is the main challenge. Data and analytics are all great if you have 80% probability and confidence in what you’re doing. If you’re in this gray zone of 50%, then it becomes difficult. That doesn’t mean you don’t use it. You do see instances where for every failure, there are many other instances of brands that are figuring it out and getting it right.
As you say that, it seemed like it was right to a lot of people. The thing is perception is such a big part of this. I write about curiosity and perception. It was fascinating to me because I remember, as I watched the ad that I was referring to, it came across as men could do better. They showed things that men have done in the past that maybe weren’t so great, whistling at women or whatever it was that they were showing. I thought, “They’re trying to make a positive thing.” To me, I didn’t see it as a negative thing. I would talk to men who got insulted by it. I started to look at it a different way. It was interesting to me how you gain that empathy by gaining curiosity if you ask questions and find out more.
I have had a lot of people on the show, even a few who have talked about creating artificial curiosity, which was fascinating to me. I remember they were doing some training and testing on computers. I can’t remember which game it was but there were different levels to the game. The computer had enough curiosity and it wanted to see what was on the next level. It is interesting to me to see that they are working on artificial means of curiosity. You wonder how much these computers are going to be able to do. You were giving examples of companies who have utilized AI in marketing and you mentioned Google. In your book, you give some examples like Google Lyft, Ancestry.com, and Coca-Cola. Do you want to share any of the stories from some of the companies you wrote about and what you thought was interesting about that?
[bctt tweet=”With the right data structure and marketing strategies, your business is destined to grow.” via=”no”]Continuing on our conversation about campaigns and missteps and perhaps successes, to me, all the examples in our book are great. It’s like choosing among my children. Given this context with what we’ve been talking about with campaigns, the Coca-Cola example is nice because it points out to one campaign that Coke did not rely on data. With Coke, the smart vending machines were their first venture. Coke sells through an intermediary and it still has a big presence through the retail channel and that’s their biggest customer. Their insight into end consumers is lower compared to direct-to-consumer brands.
I know Noodles & Company has it and many restaurants have it, which you can go and customize your Coke drink. It’s is fun for kids, even adults. What was interesting about what they did there was that they put IoTs into the smart machines and that changed the game. We talked about how you move from action to consumption and now you have a clue into consumption because when somebody walks in, you know what they’re ordering. The immediate gain from that is stockouts are a bad thing. You don’t want it. You can lose customers. You know which flavors are getting consumed in what machine and they were able to restock effectively.
Where it took the next level was Sprite Cherry. Their success in the retail stores came from data in the smart vending machines. This was the most popular combination on their smart vending machines. That’s how they knew about Sprite Cherry. They launched it into the retail services. It’s been a great hit. This is an example of how the data showed them. They couldn’t have guessed that this was the flavor people would like.
That was one example of Coke. What they did next was they took this next step into a new business model where they have Powerade. They took the smart vending machine idea to Powerade. They launched it with the Louisiana State football team. There are a lot of players on football teams. There are a lot of exercise routines. I like watching football but I don’t know what exercise routines they’re doing but it’s intense. They’re customized to different positions and players, running back and wide receiver.
Everybody has a different routine because they play different functions, and they have different days in which they do different things based on their physiology. The coaches then have to recommend drinks to the athletes or supplements that fit with the exercise routine in terms of sugar and salt levels that they need. They could use this Powerade Power Center to customize the drinks for each athlete. They could also do it in a mobile version, which could be taken to the games. In some of the LSU games, you can see it in the background, the Powerade Power Center.
That goes into how you take this whole thing about it. It starts in the consumer world. Now they’ve created a new business model where they’re customizing the whole exercise routine and supplements for football teams. You can imagine them going one team after the other now and customizing. All of this is run on understanding what people want, based on how they’re using the product, and then coming up with personalized drinks and recommendations from those insights.
That’s fascinating to me because I give a talk where I promote the value of curiosity. One of the examples I give is Ben & Jerry’s and how they look at their flavors. They get out of that status quo of thinking, “This thing is going to be great forever because it’s been great in the past.” The Sprite Cherry that you were talking about from Coke, they found that people like that. What Ben & Jerry’s did was they’d have a flavor that would be popular for a while. When it was no longer something that people wanted, instead of trying to force people to keep having it, they would give it a burial.
They have a website where they put headstones up. They recognized it, “This was successful from this year to this year. We love our success but we’re putting this one to sleep. We give them a burial.” A lot of companies try to hang on to past glory because it worked well. You then get into that status quo of Blockbuster and Kodak thing where the world passes you by. How is AI or the quantitative analysis of marketing going to help us to become more like Ben & Jerry’s or Coca-Cola?

Ben & Jerry’s is a great example. There was one company I worked with where we were doing training programs for their marketing managers. They would keep the old campaigns because they worked in the past and they had a difficult time dropping their campaign and starting new ones. A lot of companies struggle with this. This whole thing about resting on past glory is a real challenge. First of all, if you embrace this model, you’re not looking at one campaign, you’re looking at many.
What changes the mindset from the get-go is that you’re looking at a product as customizable for every individual who walks by. There is not one thing that was a big hit for many people. There is no concept of it. You are always thinking about, “For each customer that I have, and it could be my best customers or prospects, what does my brand mean for them?” What we see firms that are successful with this is they embrace the agile method of the marketing process. You adopt this process that started with product development and software development. They go into two-week agile sprints.
Whenever there is a proposal or a new campaign, it’s tested. The insights from the tests are then approved and then implemented. It’s not 3 or 6 months cycles. It’s a two-week cycle. It’s not easy to implement these. The companies that are doing that need marketing technology operations. It’s a collaborative effort. When you go down that path, it reduces your tendency to stick to past glories or things that worked in the past. The whole culture and process are meant to test and question everything that we think are the truths.
A lot of it is going out, talking to customers, doing surveys and doing things to find out what’s working and what’s not working sometimes. I’ve watched the Super Bowl for the ad. I’m one of those people. I’d love to see what the new thing is and what people find interesting. I’d love to see the feedback later. That’s probably the biggest time I would spend on Twitter to see what’s trending and what people are saying. Even with my husband, we look at certain ads. The GEICO Gecko, I don’t get it. I’ll watch that and I’m like, “Why is this popular? I don’t think it’s ever funny.” I was watching a Progressive ad where they’re like, “Don’t become your parents.” I’m thinking that’s pretty funny.
I love that one.
Isn’t that a good one? That’s great. He’s an older guy telling you not to act like an older guy, which is funny. In some of these campaigns, it’s hard to see what people are going to like. When you read about neuro-marketing and some of this stuff, they put the scanners on people’s heads, skull caps or whatever it is they do to connect them to the MRIs and all that. How accurate is the data you get from doing that thing?
You get a lot from a few customers and then it is about generalizing from that to the population. It’s the bias in AI. It is similar to that. They seem unrelated but the basic idea is that in both, are you testing on a sample that is representative of your population? Are you training your AI on a sample that is representative of the population? That is the biggest challenge in all of what we’ve talked about, even with the Nike commercial. You’re talking about curiosity and empathy.
At the end of the day, the companies need to know the target market and who they are serving. The testing, the knowledge and insights that they are gaining on a sample should be that of their target segment. When there is a mismatch, then when you launch the campaign, there is a potential for misfires. The key in any kind of data-driven marketing is yes, you need to test, but who do you test it on? Do you have enough coverage in your sample of the heterogeneity in your population? That is the main key that people tend to not grasp enough about how important that is.
[bctt tweet=”AI helps in both data and algorithms, and it really changes things.” via=”no”]There’s a lot of data we could use. As I talked to companies about building curiosity, I have some data I could point to for how we can improve engagement and curiosity. Companies like Novartis have tested it before and after doing engagement surveys and different things when they’re training people to be more curious. In marketing though, it’s a huge part of finding out what people like and whether it helps you be successful. The Ben & Jerry’s example is one, but do you get data through what you’ve been researching that shows that curiosity is helpful to building successful marketing? I want some bottom-line numbers to show that curiosity is necessary to improve productivity or income from your ad campaigns and things like that. Have you found any data in that respect?
It would be a good study to do for sure. Especially with curiosity, I don’t know where exactly the report that tests that. What I see in my world is that there was one company that talked about it that’s truth-seeking. They are truth seekers. Data-driven marketing pushes that. It is about understanding the truth about customers and your products, and being willing to accept that you were wrong in the face of new data.
That’s a hard thing for some people to give up. If it was their baby, it’s tough. Truth-seeking, getting out of status-quo thinking, whatever you want to call it, curiosity is all the same thing to me. We’re trying to get out of accepting doing things because that’s the way it’s been done without questioning.
One of the things I do in my classes to hone in on this point is yes, you want truth-seeking and curiosity, but the challenge is what’s called the confirmation bias. Managers have it and they look for yeses. You, me and everyone will have a bias to look for yeses. We need structures and processes in place, which forces us to also look for the noes, and that can help move it forward.
This is interesting because when I was writing about perception after I wrote about curiosity, what I tied it in was I thought perception is EQ, IQ, CQ, for curiosity quotient and cultural quotient. You don’t know what you don’t know. We do get a lot of confirmation bias because we do want those yeses. It’s important to look at the perception process of evaluating, predicting, interpreting, and then correlating to come up with these conclusions. As you’re working with other people, trying to put yourself in their vantage point can be challenging. To build that empathy, we’re back to curiosity.
It doesn’t matter who I talk to you about what it is. If it’s creativity, perception or whatever it is, curiosity seems to be the thing that always comes first. If you’re curious with an open mind to look for the noes, that’s a huge part of it. I found four things that inhibit curiosity and they’re fear, assumptions or the voice in your head telling you things, over and underutilization of technology, and environment, so everybody you’ve ever known.
If you analyze some of these things when you’re looking at your curiosity, then you can develop to be more open-minded and not have that sense of like, “I just want to have this because it feeds that voice in my head that makes me feel better. That’s not going to tell me the negative things.” What’s fascinating to me about studying curiosity was all the assessments told you that if you were curious or not, what your levels were. I’m like, “What if you’re low, then what do you do?” That’s why my research was to determine the things that keep you from being curious so you can move forward. For us to move forward, we need to ask those open-ended questions that maybe give us some answers that make us a little uncomfortable. How do we get more comfortable with losing what we thought we knew?
In our context with data-driven and AI, it’s not “Build it and they will come.” It costs a lot. Rightfully so, there is a lot of hesitation in senior management to invest this much with the hope that it will work. That’s why we have the five stages, foundation, experimentation, expansion, transformation and then monetization. The first stage is you need a good foundation like in building a house. That comes from data and the ability to collect first-party data. The challenge with many marketers is that direct-customer relationships and understanding directly about customers. You may not have it perfect but you might have pockets of information about your customers and you dig in there.

The second part which is appropriate for our conversation here is this notion of experimentation because you don’t know what is going to work. You’re going in with no assumptions, but you’re letting 1,000 flowers bloom and you’re looking at the ROI of all your efforts. The way to guide these decisions on what experiments to do is this concept of value pockets which we heard from one of our interviews. It’s looking at, “What can I do for my customers and my product that will improve their value proposition to consumers?” Focus on only those aspects of your product.
In my restaurant, if I change the color of my tables from white cloth to light blue cloth, that may not be great as a value pocket. If I add more reviews or encourage customers with more reviews, and if I add more activity on social media, that improves value proposition because I’m able to tell my consumers, “A lot of people like eating here and these are the things people like.” You focus on that. You use AI and data on improving your social media activities, and not on identifying the right color of tablecloth for the restaurant tables. That ends up being what we call the value pocket idea. It’s understanding what is important for your customers or to have some idea about, “These are some places where customers want some differentiation,” and then focusing your experiments on that effort. From the get-go, working on ROI and understanding where using data is helping me, and then building on that into the next steps.
In some of this stuff, I cover quite a bit in the courses that I teach still. We go over some of the examples of how companies have handled some of the things that have come up on social media, for example. I’m sure you’ve probably seen the picture that a guy had put outside his shop. It’s something like, “Come in and have the worst meatball sandwich you guys ever had on Yelp,” or whatever it is. There are a lot of things that people do to try and make the best of some bad data. It’s a challenge to come up with the hits right off the bat. Some of the people I’m talking to in my courses are in the early stages of startups. What tips can you give them to help them use AI in their marketing?
For startups and for smaller businesses, I talk about it in terms of opportunities for them because one of the things that a small business or a startup doesn’t have is legacy costs and legacy systems. With the bigger companies, one would think, “They have all the money and all the data they can work on.” It turns out, they have real problems where they cannot connect their databases. They spend a lot of time trying to do all of that before they can do any campaigns. For a startup, you don’t have that. You’re starting fresh. If you think about Netflix when it first began, it was a startup. What has helped Netflix even now is the way the data is structured and the way they’re looking at it. They have a good view of the customers. That is a real opportunity.
Many startups are in a digital space and almost every business is a direct-to-consumer digital business. To think about your business model, even if you’re not in the sense of a platform, in terms of a direct-to-consumer platform, you can build it on any cloud system. It’s mostly OpEx and not CapEx these days, so your investments are also lesser. When you start thinking about your business, think about it as, “How do I directly reach my consumers? How do I create a platform for my consumers to interact with each other? How do I become an organizer of many different services for my consumer?”
What I say to startups is to start thinking about that, and not just one product that you’re going to launch. Think about it as, “This is a beginning. How is this going to scale? How do I build my systems in a way that I can understand directly about my consumers?” That’s number one. The number two thing is that almost everybody has a website. There is Web 2.0, Instagram or YouTube. You have data about your consumers. When they’re visiting the website, you have Google Analytics. You may use HubSpot. That’s the beginning, so you can start doing it from there.
You know what web pages your customers are visiting, and what keywords are working. You can start working from that. That’s what I advise startups. One example I gave is I advised a student whose family had an Alaskan salmon fishing business. We were working on digital campaigns as part of his independent study with me. One of the things we looked at was what campaigns were working and keywords on Instagram or on Facebook. What turned out was that they were shipping 10 pounds of salmon. Hindsight is 20/20, you can understand. How many people are willing to buy 10 pounds? The minute he changed it to 5 pounds, the business took off. You knew that because you did surveys and you did different kinds of campaigns. You saw where the click-through rates and the conversion rates were high. Even if you’re a startup, you can use data to start shaping a marketing strategy.
There’s so much that you can do in any of your campaigns on Facebook and Twitter. My daughter works for a company. I don’t know if you know Split. It’s an A/B testing software on steroids. She’s a director of partnerships there. It’s fascinating to me how you can see what would work. They’ve taken it to a new level. Instead of just running this campaign and this campaign, you can do so many different versions like changing one word, changing this thing, changing that thing.
[bctt tweet=”Personalizing all aspects of your customer engagement gives you a roadmap to success.” via=”no”]My point is that the types of ways to test things now, how fascinating it’s become. She’s got so much data in her head. Some of these young startups have some great potential to get some of this data to us. It’s fascinating for achieving that maximum impact to try some of this stuff to see what’s working and what’s not. Do you think some industries are going to have more impactful results from doing that kind of thing?
Yes. Direct-to-consumer businesses are definitely going to see a lot more speed in learning using data, especially in marketing. You have consumer brands that are DTC now. FinTech and EdTech are all DTC brands. Even big or small B2B companies are all direct-to-customer. They all have a good opportunity to leverage this new data and AI. When you sell through intermediaries, you do have a challenge, but then the onus is on the brands who sell through intermediaries to develop direct relationships in other ways. In the book, we talked about Unilever and Coca-Cola as an example. It takes some effort but the end goal is directly building relationships with your end-users.
It’s going to be fun to see all the different startups and what they’re offering. Much has changed since I wrote that course based on that Publish or Perish report. I love everything that deals with marketing, especially I love the advertising thing. I think back to when I was young, “It’s time to make the donuts!” or “Where’s the beef?” I can’t believe I ate the whole thing. You name all the big campaigns at the time. The impact they have continued on. You could say some of those things to people my age and they’ll still remember.
It’s going to be fun to see how much marketing changes and advertising and everything that goes along with it in the next years. Your book is an important one, The AI Marketing Canvas, and also the Cutting Edge Marketing Analytics. You have done a lot in the field of marketing. A lot of people are going to want to know how they can find you, read your book or follow you. Is there some site or something you’d like to share?
I’m definitely on LinkedIn. That’s where I post a lot and am active. We have a website called AIMCBook.com. That’s a place where we also have a blog and continue the conversation in our book. Our book is available on Amazon.com. You can certainly reach out to me on LinkedIn. I’m happy to connect with you and continue the conversation there.
This was so great. Thank you for being on Raj. This is something that a lot of people need to know more about. I know I’ll definitely share this with my students as well. There is a lot to this that’s so important for people to get ahead of the game. Your book is timely.
Thank you, Diane. Thanks for having me. Thanks for a great conversation.
You’re welcome.
—
I’d like to thank Raj for being my guest. We get so many great guests on the show. If you’ve missed any past episodes, you can catch them at DrDianeHamilton.com. It’s a nice way to find some content if you don’t have time to read the entire show. I hope you take some time to explore the site. Please notice at the top, you have the curiosity and perception drop-down menu. If you want to look at the Curiosity Code Index or if you want to look at the Perception Power Index, it’s all there. I hope you enjoyed this episode. I hope you join us for the next episode of Take The Lead Radio.
Important Links:
- Rajkumar Venkatesan
- The AI Marketing Canvas
- Marketing Analytics
- Publish Or Perish – Forbes article
- Bruce Rogers – past episode
- Ancestry.com
- Split
- LinkedIn – Rajkumar Venkatesan, Ph.D.
- Amazon.com – The AI Marketing Canvas
- Curiosity Code Index
- Perception Power Index
- Cutting Edge Marketing Analytics
About Rajkumar Venkatesan
Rajkumar Venkatesan is the Ronald Trzcinski Professor of Business Administration at the Darden Business School at the University of Virginia. Raj has written about and taught quantitative marketing to MBA and executive education students worldwide. At Darden, he has taught a course on marketing analytics for more than ten years, and a course on marketing technology products for five years.
His experience in these courses he translated into the books, Cutting Edge Marketing Analytics, published by Pearson Education in 2014, and The AI Marketing Canvas in 2021. He has published extensively in the Journal of Marketing, Journal of Marketing Research, Marketing Science, Journal of Academy of Marketing Science, International Journal of Research in Marketing, Harvard Business Review, and California Management Review.
He serves as an Associate Editor for Journal of Marketing, International Journal of Research in Marketing, and Journal of Academy of Marketing Science. More than 250,000 individuals have participated in his Coursera course on marketing analytics.
Love the show? Subscribe, rate, review, and share!
Join the Take The Lead community today: